Boosting, especially of decision trees, is among the most prevalent and powerful machine learning algorithms.
There are many variants of boosting algorithms and frameworks implementing those algorithms. XGBoost—short for the exciting moniker extreme gradient boosting—is one of the most well-known algorithms with an accompanying, and even more popular, framework.
This article will guide you through the nuances of XGBoost (the algorithm) and how to use XGBoost (the framework).
*Looking for the Colab Notebook for this post? Find it right here.*
What is XGBoost?
The term “XGBoost” can refer to both a gradient boosting algorithm for decision trees that solves many data science problems in a fast and accurate way and an open-source framework implementing that algorithm.
To disambiguate between the two meanings of XGBoost, we’ll call the algorithm “XGBoost the Algorithm” and the framework “XGBoost the Framework.”
To understand XGBoost the Framework, we first have to understand XGBoost the Algorithm.
What is XGBoost the Algorithm?
As the name may reveal, XGBoost the Algorithm is a gradient boosting algorithm, a common technique in ensemble learning.
To unpack that new phrase, ensemble learning is a type of machine learning that enlists many models to make predictions together. Boosting algorithms are distinguished from other ensemble learning techniques by building a sequence of initially weak models into increasingly more powerful models. Gradient boosting algorithms choose how to build a more powerful model using the gradient of a loss function that captures the performance of a model.
Gradient boosting is a foundational approach to many machine learning algorithms. XGBoost has solidified its name in the boosting game with its use in many competition-winning models and prolific reference in research.
How does XGBoost the Algorithm work?
XGBoost the Algorithm operates on decision trees, models that construct a graph that examines the input under various “if” statements (vertices in the graph). Whether the “if” condition is satisfied influences the next “if” condition and eventual prediction. XGBoost the Algorithm progressively adds more and more “if” conditions to the decision tree to build a stronger model.
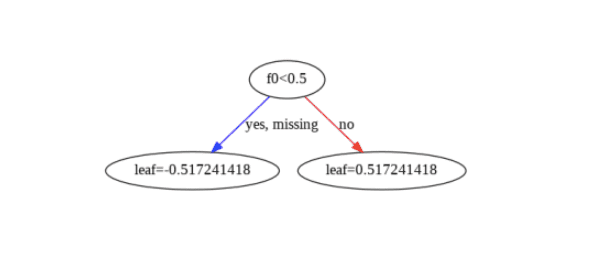
How does XGBoost the Algorithm work?
XGBoost the Framework implements XGBoost the Algorithm and other generic gradient boosting techniques for decision trees.
XGBoost the Framework is maintained by open-source contributors—it’s available in Python, R, Java, Ruby, Swift, Julia, C, and C++ along with other community-built, non-official support in many other languages.
XGBoost the Algorithm was first published by University of Washington researchers in 2016 as a novel gradient boosting algorithm. Like other gradient boosting algorithms on decision trees, XGBoost considers the leaves of the current decision tree and questions whether turning that leaf into a new “if” statement with separate predictions would benefit the model. The benefit to the model depends on the “if” statement chosen and which leaf it’s placed on—this can be determined using the gradient of the loss. The loss includes a scoring function that measures algorithm performance.
Get To Know Other Data Science Students
Sunil Ayyappan
Senior Technical Program Manager (AI) at LinkedIn
Abby Morgan
Data Scientist at NPD Group
Nick Lenczewski
Data Scientist at Ovative Group
What sets XGBoost the Algorithm apart?
XGBoost the Algorithm sets itself apart from other gradient boosting techniques by using a second-order approximation of the scoring function. This approximation allows XGBoost to calculate the optimal “if” condition and its impact on performance. XGBoost The Algorithm can then store these in its memory the next decision tree to save recomputing it.
XGBoost the Algorithm is powerful on its own but is also a great fixer-upper using the other tools from your machine learning toolbox. Consider feature engineering for instance, where the machine learning engineer preprocesses the raw inputs into new input features before letting the model get its hands dirty. XGBoost the Algorithm makes the most of engineered features and can produce a nicely interpretable and high performing model.
How do you use XGBoost?
Together, XGBoost the Algorithm and XGBoost the Framework form a great pairing with many uses.
- XGBoost the Algorithm learns a model faster than many other machine learning models and works well on categorical data and limited datasets.
- XGBoost the Framework is highly efficient and developer-friendly and extremely popular among the data scientists community with lots of documentation and online support.
These advantages make XGBoost (both the algorithm and the framework) useful for many machine learning applications.
If you’d like to learn more and begin using XGBoost like a professional, check out Springboard’s Machine Learning Career Track.
Companies are no longer just collecting data. They’re seeking to use it to outpace competitors, especially with the rise of AI and advanced analytics techniques. Between organizations and these techniques are the data scientists – the experts who crunch numbers and translate them into actionable strategies. The future, it seems, belongs to those who can decipher the story hidden within the data, making the role of data scientists more important than ever.
In this article, we’ll look at 13 careers in data science, analyzing the roles and responsibilities and how to land that specific job in the best way. Whether you’re more drawn out to the creative side or interested in the strategy planning part of data architecture, there’s a niche for you.
Is Data Science A Good Career?
Yes. Besides being a field that comes with competitive salaries, the demand for data scientists continues to increase as they have an enormous impact on their organizations. It’s an interdisciplinary field that keeps the work varied and interesting.
10 Data Science Careers To Consider
Whether you want to change careers or land your first job in the field, here are 13 of the most lucrative data science careers to consider.
Data Scientist
Data scientists represent the foundation of the data science department. At the core of their role is the ability to analyze and interpret complex digital data, such as usage statistics, sales figures, logistics, or market research – all depending on the field they operate in.
They combine their computer science, statistics, and mathematics expertise to process and model data, then interpret the outcomes to create actionable plans for companies.
General Requirements
A data scientist’s career starts with a solid mathematical foundation, whether it’s interpreting the results of an A/B test or optimizing a marketing campaign. Data scientists should have programming expertise (primarily in Python and R) and strong data manipulation skills.
Although a university degree is not always required beyond their on-the-job experience, data scientists need a bunch of data science courses and certifications that demonstrate their expertise and willingness to learn.
Average Salary
The average salary of a data scientist in the US is $156,363 per year.
Data Analyst
A data analyst explores the nitty-gritty of data to uncover patterns, trends, and insights that are not always immediately apparent. They collect, process, and perform statistical analysis on large datasets and translate numbers and data to inform business decisions.
A typical day in their life can involve using tools like Excel or SQL and more advanced reporting tools like Power BI or Tableau to create dashboards and reports or visualize data for stakeholders. With that in mind, they have a unique skill set that allows them to act as a bridge between an organization’s technical and business sides.
General Requirements
To become a data analyst, you should have basic programming skills and proficiency in several data analysis tools. A lot of data analysts turn to specialized courses or data science bootcamps to acquire these skills.
For example, Coursera offers courses like Google’s Data Analytics Professional Certificate or IBM’s Data Analyst Professional Certificate, which are well-regarded in the industry. A bachelor’s degree in fields like computer science, statistics, or economics is standard, but many data analysts also come from diverse backgrounds like business, finance, or even social sciences.
Average Salary
The average base salary of a data analyst is $76,892 per year.
Business Analyst
Business analysts often have an essential role in an organization, driving change and improvement. That’s because their main role is to understand business challenges and needs and translate them into solutions through data analysis, process improvement, or resource allocation.
A typical day as a business analyst involves conducting market analysis, assessing business processes, or developing strategies to address areas of improvement. They use a variety of tools and methodologies, like SWOT analysis, to evaluate business models and their integration with technology.
General Requirements
Business analysts often have related degrees, such as BAs in Business Administration, Computer Science, or IT. Some roles might require or favor a master’s degree, especially in more complex industries or corporate environments.
Employers also value a business analyst’s knowledge of project management principles like Agile or Scrum and the ability to think critically and make well-informed decisions.
Average Salary
A business analyst can earn an average of $84,435 per year.
Database Administrator
The role of a database administrator is multifaceted. Their responsibilities include managing an organization’s database servers and application tools.
A DBA manages, backs up, and secures the data, making sure the database is available to all the necessary users and is performing correctly. They are also responsible for setting up user accounts and regulating access to the database. DBAs need to stay updated with the latest trends in database management and seek ways to improve database performance and capacity. As such, they collaborate closely with IT and database programmers.
General Requirements
Becoming a database administrator typically requires a solid educational foundation, such as a BA degree in data science-related fields. Nonetheless, it’s not all about the degree because real-world skills matter a lot. Aspiring database administrators should learn database languages, with SQL being the key player. They should also get their hands dirty with popular database systems like Oracle and Microsoft SQL Server.
Average Salary
Database administrators earn an average salary of $77,391 annually.
Data Engineer
Successful data engineers construct and maintain the infrastructure that allows the data to flow seamlessly. Besides understanding data ecosystems on the day-to-day, they build and oversee the pipelines that gather data from various sources so as to make data more accessible for those who need to analyze it (e.g., data analysts).
General Requirements
Data engineering is a role that demands not just technical expertise in tools like SQL, Python, and Hadoop but also a creative problem-solving approach to tackle the complex challenges of managing massive amounts of data efficiently.
Usually, employers look for credentials like university degrees or advanced data science courses and bootcamps.
Average Salary
Data engineers earn a whooping average salary of $125,180 per year.
Database Architect
A database architect’s main responsibility involves designing the entire blueprint of a data management system, much like an architect who sketches the plan for a building. They lay down the groundwork for an efficient and scalable data infrastructure.
Their day-to-day work is a fascinating mix of big-picture thinking and intricate detail management. They decide how to store, consume, integrate, and manage data by different business systems.
General Requirements
If you’re aiming to excel as a database architect but don’t necessarily want to pursue a degree, you could start honing your technical skills. Become proficient in database systems like MySQL or Oracle, and learn data modeling tools like ERwin. Don’t forget programming languages – SQL, Python, or Java.
If you want to take it one step further, pursue a credential like the Certified Data Management Professional (CDMP) or the Data Science Bootcamp by Springboard.
Average Salary
Data architecture is a very lucrative career. A database architect can earn an average of $165,383 per year.
Machine Learning Engineer
A machine learning engineer experiments with various machine learning models and algorithms, fine-tuning them for specific tasks like image recognition, natural language processing, or predictive analytics. Machine learning engineers also collaborate closely with data scientists and analysts to understand the requirements and limitations of data and translate these insights into solutions.
General Requirements
As a rule of thumb, machine learning engineers must be proficient in programming languages like Python or Java, and be familiar with machine learning frameworks like TensorFlow or PyTorch. To successfully pursue this career, you can either choose to undergo a degree or enroll in courses and follow a self-study approach.
Average Salary
Depending heavily on the company’s size, machine learning engineers can earn between $125K and $187K per year, one of the highest-paying AI careers.
Quantitative Analyst
Qualitative analysts are essential for financial institutions, where they apply mathematical and statistical methods to analyze financial markets and assess risks. They are the brains behind complex models that predict market trends, evaluate investment strategies, and assist in making informed financial decisions.
They often deal with derivatives pricing, algorithmic trading, and risk management strategies, requiring a deep understanding of both finance and mathematics.
General Requirements
This data science role demands strong analytical skills, proficiency in mathematics and statistics, and a good grasp of financial theory. It always helps if you come from a finance-related background.
Average Salary
A quantitative analyst earns an average of $173,307 per year.
Data Mining Specialist
A data mining specialist uses their statistics and machine learning expertise to reveal patterns and insights that can solve problems. They swift through huge amounts of data, applying algorithms and data mining techniques to identify correlations and anomalies. In addition to these, data mining specialists are also essential for organizations to predict future trends and behaviors.
General Requirements
If you want to land a career in data mining, you should possess a degree or have a solid background in computer science, statistics, or a related field.
Average Salary
Data mining specialists earn $109,023 per year.
Data Visualisation Engineer
Data visualisation engineers specialize in transforming data into visually appealing graphical representations, much like a data storyteller. A big part of their day involves working with data analysts and business teams to understand the data’s context.
General Requirements
Data visualization engineers need a strong foundation in data analysis and be proficient in programming languages often used in data visualization, such as JavaScript, Python, or R. A valuable addition to their already-existing experience is a bit of expertise in design principles to allow them to create visualizations.
Average Salary
The average annual pay of a data visualization engineer is $103,031.
Resources To Find Data Science Jobs
The key to finding a good data science job is knowing where to look without procrastinating. To make sure you leverage the right platforms, read on.
Job Boards
When hunting for data science jobs, both niche job boards and general ones can be treasure troves of opportunity.
Niche boards are created specifically for data science and related fields, offering listings that cut through the noise of broader job markets. Meanwhile, general job boards can have hidden gems and opportunities.
Online Communities
Spend time on platforms like Slack, Discord, GitHub, or IndieHackers, as they are a space to share knowledge, collaborate on projects, and find job openings posted by community members.
Network And LinkedIn
Don’t forget about socials like LinkedIn or Twitter. The LinkedIn Jobs section, in particular, is a useful resource, offering a wide range of opportunities and the ability to directly reach out to hiring managers or apply for positions. Just make sure not to apply through the “Easy Apply” options, as you’ll be competing with thousands of applicants who bring nothing unique to the table.
FAQs about Data Science Careers
We answer your most frequently asked questions.
Do I Need A Degree For Data Science?
A degree is not a set-in-stone requirement to become a data scientist. It’s true many data scientists hold a BA’s or MA’s degree, but these just provide foundational knowledge. It’s up to you to pursue further education through courses or bootcamps or work on projects that enhance your expertise. What matters most is your ability to demonstrate proficiency in data science concepts and tools.
Does Data Science Need Coding?
Yes. Coding is essential for data manipulation and analysis, especially knowledge of programming languages like Python and R.
Is Data Science A Lot Of Math?
It depends on the career you want to pursue. Data science involves quite a lot of math, particularly in areas like statistics, probability, and linear algebra.
What Skills Do You Need To Land an Entry-Level Data Science Position?
To land an entry-level job in data science, you should be proficient in several areas. As mentioned above, knowledge of programming languages is essential, and you should also have a good understanding of statistical analysis and machine learning. Soft skills are equally valuable, so make sure you’re acing problem-solving, critical thinking, and effective communication.
Since you’re here…Are you interested in this career track? Investigate with our free guide to what a data professional actually does. When you’re ready to build a CV that will make hiring managers melt, join our Data Science Bootcamp which will help you land a job or your tuition back!